Unlocking the Power of Image Labeling: A Game-Changer for Classification
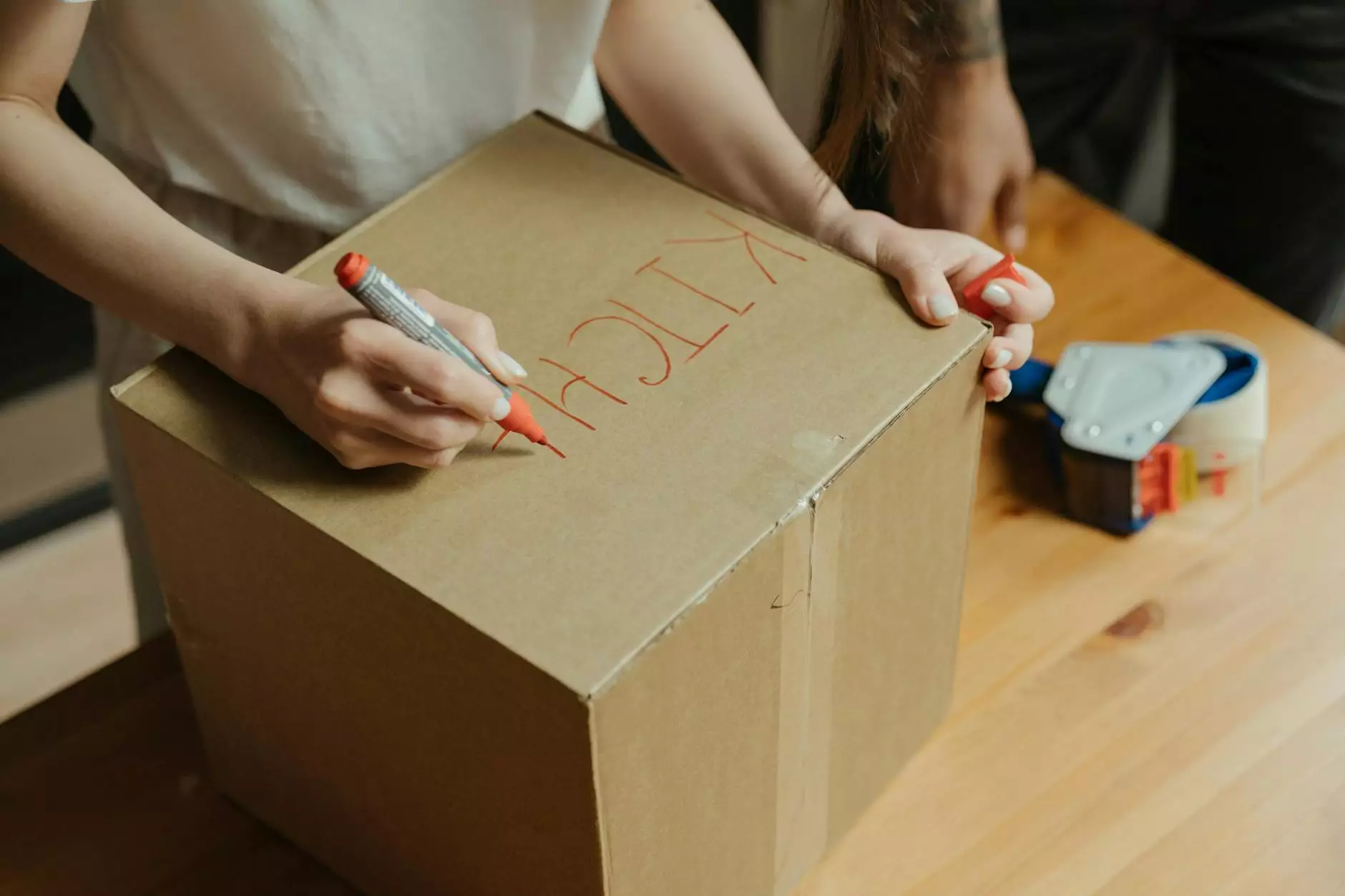
Introduction to Image Labeling and Its Importance
Image labeling tools have become a pivotal part of the data annotation landscape, especially for businesses focused on machine learning and artificial intelligence. In a world where visual data is rapidly increasing, the need for efficient classification methods is paramount.
In essence, an image labeling tool for classification allows organizations to transform unstructured image data into structured datasets that can be easily interpreted by algorithms. This process not only supports machine learning applications but also enhances decision-making processes across various domains.
The Role of Data Annotation in Machine Learning
Data annotation is the method of labeling data to train machine learning models. This process is crucial because the performance of a model greatly depends on the quality of its training data. When it comes to images, the classification tasks can range from simple object detection to complex scene recognition.
Utilizing an image labeling tool for classification ensures that your data is accurately labeled, improving the overall effectiveness of your models. Let's dive deeper into why having a dedicated tool for this purpose is essential.
Key Benefits of Using an Image Labeling Tool
When organizations implement an image labeling tool for classification, they can expect a plethora of benefits, including:
- Improved Accuracy: Manual labeling can often lead to inconsistencies and errors. A dedicated tool provides automation features that enhance accuracy.
- Efficiency: Automated labeling reduces the time required for data annotation, allowing teams to focus on model training and improvement.
- Scalability: As data volumes grow, tools provide scalable solutions that can handle large datasets without compromising quality.
- Collaboration: Many tools offer features for multiple users, enabling teams to collaborate effectively on data annotation projects.
- Cost-Effectiveness: While investing in a labeling tool may seem costly initially, the long-term savings, resulting from increased productivity and reduced errors, prove beneficial.
How to Choose the Right Image Labeling Tool
With numerous options available, selecting the ideal image labeling tool for classification can be daunting. Here are key aspects to consider:
User-Friendliness
The interface should be intuitive and easy to navigate, allowing users to start labeling images quickly.
Supported Formats
Ensure the tool can support various image formats (JPEG, PNG, etc.) and is compatible with your existing systems.
Automation Features
Look for tools that incorporate AI-driven automation features to assist during the labeling process, ensuring speed and accuracy.
Importing and Exporting Data Capabilities
Check if the tool allows for seamless importing of images and exporting of labeled data in formats compatible with your machine learning framework.
Cost Considerations
Evaluate the pricing models of different tools. Some may offer subscription models, while others might charge per use or provide one-time purchase options.
Support and Training
Consider whether the provider offers adequate training and support to help your team get started.
Integrating Image Labeling Tools with Machine Learning Platforms
Once you've selected the right image labeling tool for classification, the next step involves integrating it with your machine learning platform. This integration facilitates a smooth workflow from data labeling to model training.
Consider the following best practices for integration:
- API Connectivity: Use tools with robust API support for easy integration into your existing tech stack.
- Real-Time Data Sync: Opt for solutions that support real-time data synchronization to maintain up-to-date datasets.
- Testing and Validation: After integration, conduct rigorous testing to ensure the labeled data meets the model's requirements.
- Feedback Loop: Create a feedback mechanism to continually improve the labeling process based on model performance.
Case Studies: Success Stories with Image Labeling Tools
To exemplify the effectiveness of using an image labeling tool for classification, let's look at a few case studies:
Case Study 1: Healthcare Imaging
In the healthcare sector, a leading medical imaging company used a sophisticated image labeling tool to classify thousands of MRI scans. The automated tool enabled the annotation of images with high accuracy, significantly reducing human error. As a result, the company improved the diagnostic process and reduced patient waiting times.
Case Study 2: Retail Analytics
A major retail brand implemented an image labeling tool to identify products in their inventory management system. By classifying images of products accurately and efficiently, the retailer enhanced its inventory tracking and improved supply chain optimization, leading to a noticeable increase in sales.
Case Study 3: Autonomous Vehicles
In the automotive industry, a developer of autonomous vehicle technology utilized an image labeling tool to classify various road signs, pedestrian types, and environmental conditions from image data. This effort was essential for training their self-driving algorithms, resulting in a safer and more reliable driving experience.
Final Thoughts: Embrace the Future of Data Annotation
The demand for an effective image labeling tool for classification will only continue to grow as machine learning technologies advance. Organizations that invest in these tools and integrate them within their frameworks will undoubtedly unlock vast potentials.
By understanding the nuances of data annotation, businesses can leverage high-quality training data to enhance their models and gain a competitive edge. With the right strategies and tools in place, your organization can efficiently transform raw image data into actionable insights, driving success in your respective industry.
For more information on data annotation tools and platforms, visit KeyLabs.ai.